By Dr. William Oliver Hedgepath | 12/24/2024
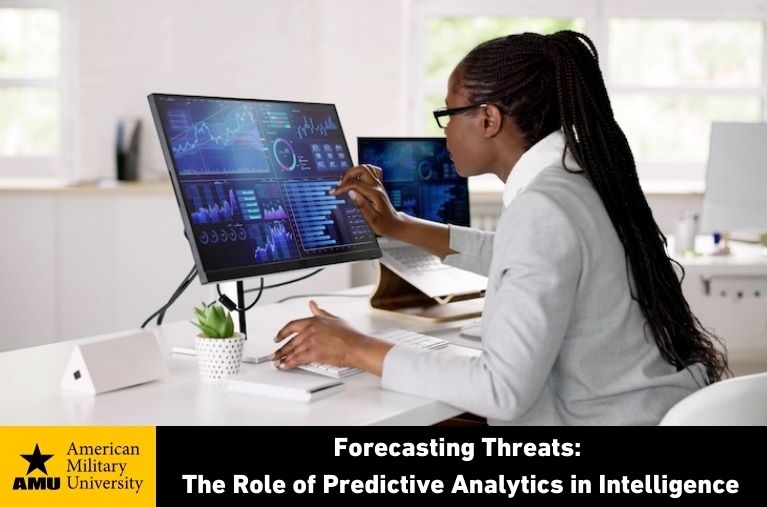
Predictive analytics plays a crucial role in forecasting and mitigating security threats in today's complex, busy world. This field combines mathematics, statistics, and advanced technology to uncover patterns and trends that drive informed decision-making.
The Evolution of Predictive Analytics
My journey with predictive analytics began during my career with the Defense Intelligence Agency (DIA). Over time, my role evolved from mathematician to operations research systems analyst, also known as an OR/SA.
The OR/SA position was a product born out of World War II (WWII) when there was a need to determine potential military threats and avoid conflict. Today, security professionals in the Department of Defense (DoD) or the intelligence agencies might hold titles other than OR/SA. OR/SA has expanded to include:
- Data scientists
- Machine learning engineers
- AI specialists
- Predictive analytics experts
These job titles reflect the integration of advanced data processing technologies such as artificial intelligence (AI) and machine learning (ML) tools. These technologies are capable of processing huge amounts of data and can significantly enhance the accuracy of data-driven decisions in regard to potential security threats.
What Are Predictive Analytics?
Predictive analytics uses data, math, and technology to predict what events might happen next. Whether predictive analytics is used for identifying potential risks or spotting trends, the work of researchers and analysts help intelligence organizations to make smarter, data-driven decisions for the prevention of attacks.
Predictive analytics tools can be as simple as a basic spreadsheet or as advanced as cutting-edge data visualization software. Analysts often dive into historical data – sometimes spanning months, years, or even decades – to uncover patterns and trends that inform smart decisions. By blending data-driven insights with the latest information provided by AI technology tools, predictive analytics has changed the way intelligence organizations approach threat forecasting.
The Components of Predictive Analytics
Predictive analytics relies on several components, including:
- Relevant data: The foundation of any analysis, encompassing both current and historical information
- Statistical algorithms: Advanced mathematical formulas for analyzing data and drawing meaningful conclusions
- Basic arithmetic and statistics: Essential tools for identifying and quantifying patterns and trends that could identify future threats
These components of predictive analytics can be used by analysts to pinpoint a particular security threat. For instance, imagine a war-torn country where terrorist acts frequently occur. Predictive analytics could be used to pinpoint where the greatest number of terrorist attacks are occurring so that leaders could more easily determine where people and tools for maintaining security should be sent.
Real-World Applications of Predictive Analytics
Predictive analytics is used to anticipate possible types of security threats. In recent years, attacks have included vulnerable targets such as:
- Civilians, especially events with many people
- Military and government buildings
- Non-military buildings such as shopping malls, schools, places of worship, and movie theaters
- Computer systems
- International airports, train stations, and buses
- High-profile landmarks, which attackers often see as symbolic
- Critical infrastructure
Terrorist attacks can originate from individuals and organizations, either inside the U.S. or from foreign countries. To anticipate and mitigate potential future incidents that could affect our national defense or homeland security, it is essential to employ predictive models, visual aids, and virtual displays.
How Federal Agencies Leverage Predictive Analytics
In organizations such as the DIA, Central Intelligence Agency (CIA), or the Federal Bureau of Investigation (FBI), a predictive analysis is an essential and crucial tool they use to enhance their security practices and security posture. However, each organization has unique missions and focus for forecasting threats.
The DIA mainly supports data analysis for military operations and strategic planning for the Department of Defense (DoD). The DIA uses predictive analytics for various reasons:
- To identify current and future threats to military operations or personnel
- To examine an adversary’s potential threat capabilities or attack methods (soldiers or weapons of war)
- To address the ever-growing number of attackers
By analyzing information, DIA can then offer valuable insights to military leaders, the Pentagon, and the President of the United States for strategic decision-making.
In my time at the DIA, these analytics often involved structured analytics techniques (SAT). SAT analysis involves evaluating and proposing scenarios for a potential threat, as well as looking at and relying on trends in threat and relevant data.
CIA has similar missions to the DIA. The FBI also investigates the same kinds of data sources, but it has a greater level of local support to fight against security threats, especially terroristic threats and acts.
Working with Complex Data Sets
“Relevant data,” although it is a common term, has complex implications. The data points that intelligence agencies typically gather comes from a variety of threat intelligence sources, such as:
- Images, troop movements, and other information captured by satellites, ships, ground sites, or planes, involving imagery intelligence (IMINT) and measurement and signatures intelligence (MASINT)
- Signals intelligence (SIGINT), which comes from capturing electronic transmissions
- Human intelligence (HUMINT), primarily involving information from people, personality profiles, and financial records
- Open source intelligence (OSINT), consisting of knowledge gathered from public sources such as news articles, social media sites, websites, and public records
Ensuring Data Accuracy
Before using data, analysts must review whether it is credible. If data collection methods or the data itself are not accurate, forecasting a potential threat such as a terroristic act might lead to the failure of any proposed response to address that threat. In addition, the amount of data has increased over the past decades with the advent of social media.
The RAND Corporation®, a nonprofit research organization that supplies leaders with useful information for making evidence-based decisions, created a concept called Assumption-Based Planning (ABP). ABP is an approach to examining the uncertainty tied to collected data.
Using ABP methodology, each data point needs to include a clearly defined assumption of its accuracy. For every piece of data, there should be an explicit statement about how reliable or precise it is, allowing analysts to understand potential uncertainties or biases and factor them into their evaluations and decisions.
Each assumption for each piece of data must be evaluated for any vulnerabilities or risks of inaccuracy. These assumptions and vulnerabilities are also linked to another key part of data validity – time.
For example, if data collection has occurred over the last three months or three years, is that data still valid today? ABP also examines the human and environmental factors to help assess each piece of data’s usefulness for the current predictive analytics of a threat.
Despite these challenges, however, predictive analytics continues to prove invaluable, offering insights that help intelligence organizations anticipate and adapt to ever-changing threats.
Data Collection Challenges
Collecting accurate and reliable data certainly has its challenges, especially when it involves threat intelligence. Disinformation or misleading information delivered through the media continues to be an issue, because it raises concerns over what data is true or false. Disinformation intentionally spreads false or misleading information, which can contaminate the datasets that predictive models rely on.
When analysts process flawed data, they are more likely to produce false positives or false negatives. As a result, this disinformation taints the data and leads to inaccurate predictions that further erode confidence and result in misguided or even harmful actions by decision makers.
Privacy is another challenge when it comes to collecting data from people for threat forecasting. When data is collected from people (for example, through interviews or surveys), privacy becomes a big concern. If the data is not handled carefully, its exposure could embarrass individuals or even put their safety at risk.
Ideally, security measures should be put in place to protect people's information. If data is highly sensitive (as in top secret government information), there should be security controls in place.
AI and Machine Learning in Threat Forecasting
While AI is useful in helping to collect data, sorting unstructured big data, and creating analyses, it is imperative to stress that the human element is more important than relying only on AI tools when it comes to today's threat landscape. Human interpretation is key to successfully using analytical methods for threat forecasting and determining what vulnerable areas need safeguarding.
Predictive analytics can also involve using statistical techniques and machine learning algorithms to analyze historical data and make predictions about future events. When combined with text-mining software tools, predictive analytics can extract valuable insights from unstructured text data, such as customer reviews, social media posts, and survey responses.
One analytical tool is Leximancer® – a text-mining software that analyzes concepts and words to create a visual map or a connection between words and concepts. These visual maps provide an analyst with a unique view and a potentially greater understanding of the underlying themes – or more importantly – patterns between words, concepts, and threats. However, it’s important to note that there is a distinction between individual words and the broader concepts they represent, especially when defining and understanding threats.
Imagine a concept map where each of these threats is a central node, with the keywords branching out from them. Words that frequently appear together in the text would be grouped into the same concept, showing their relationships and connections.
Here's an example of a visual map:
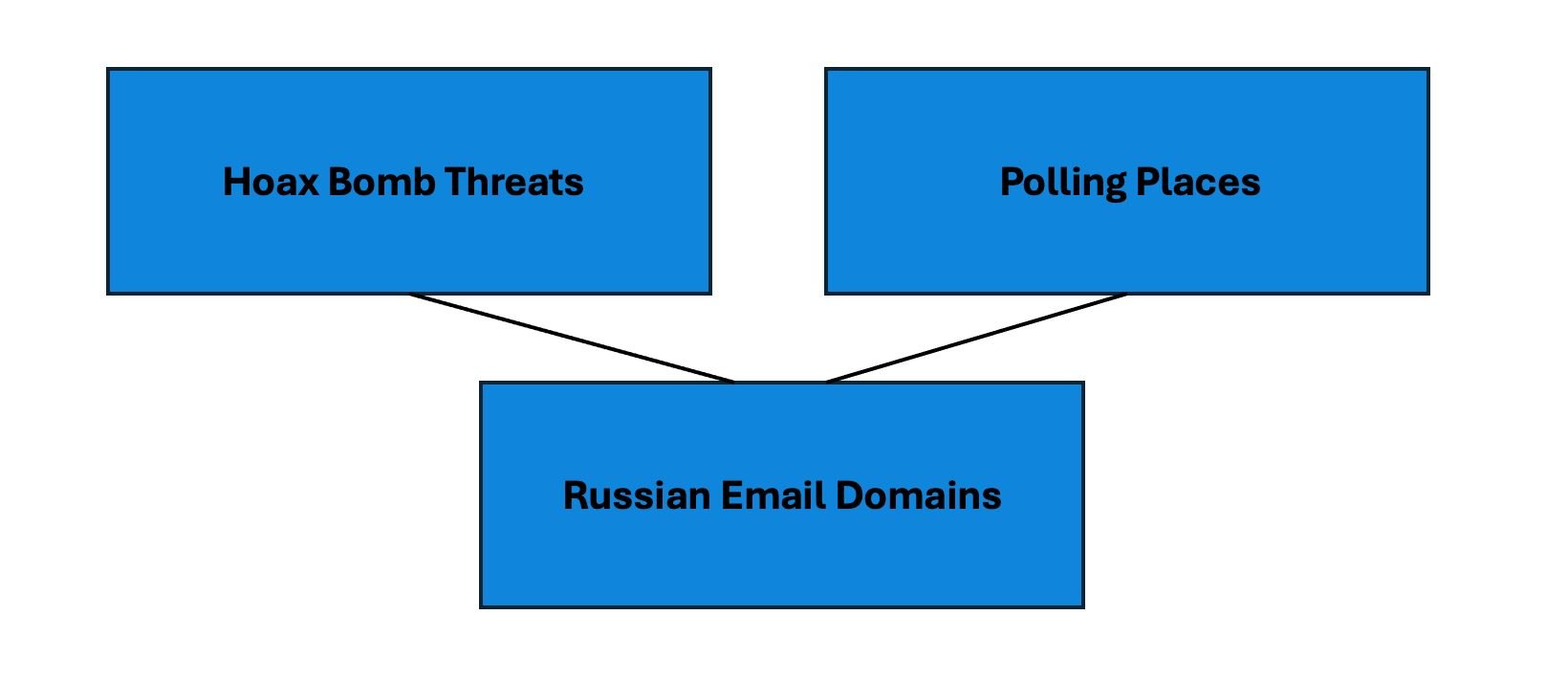
A visual map forecasting threats that could become a reality. Image courtesy of author.
This type of visual map is useful in forecasting possible security threats from attackers. It shows the connection between the words “Hoax Bomb Threat,” “Polling Places,” and “Russian Email Domains.”
This type of analysis provides security personnel with a blueprint of critical vulnerabilities and also serves as a starting point for discussions about emerging threats and the exploration of potential solutions.
The evolving nature of attacks – such as cyber data breaches and terrorist attacks – requires continuous data gathering to proactively identify threats and vulnerabilities. As a result, data gathering and threat forecasting can be expensive and time-consuming.
As predictive analytics continues to evolve, the possibilities for innovation are endless. Advancements in AI, machine learning, and data visualization redefine the way we tackle intelligence collection challenges in national security. The future of predictive analytics is one we’re only beginning to explore.
Intelligence Degrees at American Military University
For students interested in predictive analytics, threat forecasting, the Intelligence Community, and other areas of intelligence, American Military University (AMU), offers two degrees:
- An online bachelor’s degree in intelligence studies
- An online master’s degree in intelligence studies
Courses in these degree programs include intelligence collection, critical analysis, threat analysis, and foreign intelligence organizations. Other courses include strategic intelligence, intelligence analysis, and ethical challenges in the Intelligence Community. All of these courses are taught by faculty members with a deep knowledge of the intelligence field.
For more details on these degree programs, visit our intelligence degrees program page.
The RAND Corporation is a registered trademark of The RAND Corporation.
Leximancer is a registered trademark of UniQuest Pty, Ltd.
Dr. Oliver Hedgepeth is a full-time professor at the Dr. Wallace E. Boston School of Business. He teaches and publishes on reverse logistics as well as transportation and logistics. Dr. Hedgepeth holds a bachelor’s degree in chemistry from Barton College, a master’s degree in engineering management from Old Dominion University and a Ph.D. in engineering management from Old Dominion University.
Before his teaching career, Dr. Hedgepeth was an operations research systems analyst for the Department of Defense (DOD) and the Defense Intelligence Agency (DIA). He was an active member of the Military Operations Research Society (MORS) and had many articles published in Phalanx, their magazine used by professionals in DoD and Department of Homeland Security (DHS) and government contractors.